FINANCE
5 AI TRENDS PROFOUNDLY BENEFITING BUSINESS BOTTOM LINES
Expert cites machine learning advancements creating immediate, actionable value to drive data literacy, elevate cognitive insights and increase profitability in kind.
MERILEE KEM, MBA
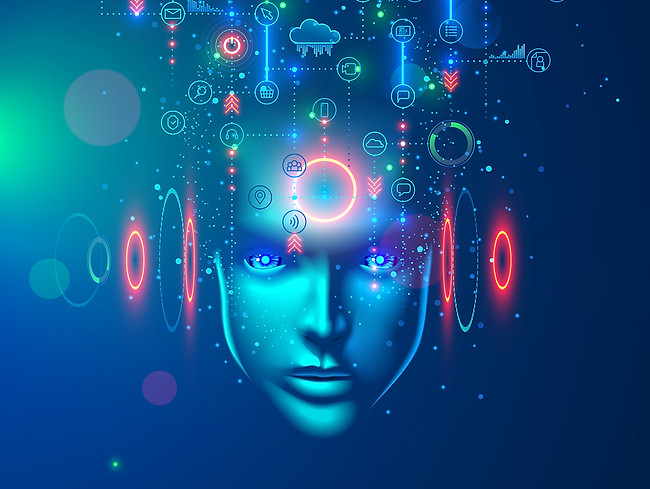
I n today’s tumultuous business-scape amid increasingly complex and often vexing marketplace conditions, curating and mining data to drive analytics-based decision making is just no longer enough. For competing with maximum, sustained impact and mitigated opportunity loss, it’s rapidly monetizing data that’s now the name of the game—particularly when spurred by artificial intelligence (AI). Indeed, emerging AI methodologies help forward-thinking companies achieve and sustain true agility, fuel growth, and compete far more aggressively than ever before.
AI is critical to those ends and certainly for aptly predicting, preparing, and responding to future crises as with the COVID-19 pandemic. Gartner recently cited the need for “smarter, faster, more responsible AI” as its No. 1 top trend that data and analytics leaders should focus on—particularly those looking to “make essential investments to prepare for a post-pandemic reset.” Novel coronavirus matters aside, Gartner underscored just how impactful AI would become, predicting that, “by the end of 2024, 75% of enterprises will shift from piloting to operationalizing AI, driving a 5X increase in streaming data and analytics infrastructures.”
“To innovate their way beyond the post-COVID-19 world, data and analytics leaders require an ever-increasing velocity and scale of analysis in terms of processing and access to succeed in the face of unprecedented market shifts,” said Rita Sallam, Distinguished VP Analyst, Gartner.
However, employing AI techniques like Machine Learning (ML) and Natural Language Processing (NLP) to glean insights and render projections is simply no longer “enough” to get the job done— especially for organizations seeking to compete efficiently on a national, multi-national or global scale. Today’s organizations must endeavor toward a culture of AI-driven data literacy that directly and positively influences their top and bottom lines.
“To help data monetization-minded enterprises better future-proof their operations and asset-amplify their data value chain. There are a few key ways to implement and elevate machine intelligence so that it’s far smarter, faster, and more accountable than protocols past,” said Microsoft alum Irfan Khan, founder, and CEO of CLOUDSUFI—an AI solutions firm automating data supply chains to propel and actualize data monetization.
Below, Khan details five benefits of leveraging AI data-driven insights and technology in a way that will create actual and actionable value right now—the kind of insights that enable new and evolved business models and empower companies to increase both revenue and profitability.

Manifesting New Market Opportunities
Today’s machine learning capabilities allow people to sift through data that previously could not be accessed, all at speeds faster than ever before. Present technology offers the opportunity to wholly analyze images, spoken, or written inputs rather than just numerical, helping companies better find connections across these diverse data sets. This generates and maximizes value in a number of ways. Relative to the bottom and top lines, not only can it significantly reduce expenses, but it can also create new market opportunities. With COVID-19 as one recent example, algorithms speedily sifted through an extraordinary amount of data to identify diseases and potential cures that presented as similar, which allowed those methodologies to be readily tested against the coronavirus.
Machine learning advancements also help companies better monetize their data and establish new revenue streams. In the above example, of course, patient information would not be shared or sold in any way, but other highly valuable data points can be gleaned. This includes determining that a certain drug is only effective on women between certain ages—critical insights for pharmaceutical developers and physicians.
Emerging AI data processing protocols are far more rapid than prior iterations of machine learning technology, resulting in the resulting solutions, discoveries, and profit-producing results.
Reconcile Emotions with Actualities
Data generates value, which leads to the generation of money. It’s that simple. Previously, it was difficult, if not humanly impossible, to sift through mass amounts of data and pinpoint relationships. There existed very rudimentary tools like regression and correlation, but today’s analytics call for gaining a proper understanding of what extracted data means. How do you convert data into a story you can tell? Often, decisions are made based on emotional foundations. Leaders are using data to either validate their gut or disagree with their instincts. Now, they are getting quicker insights that decisively validate or invalidate their thinking while also prompting them to ask new questions. So, garnering meaning out of a company’s data provides tremendous advantages.
“Human nature is such that unless we can see it, touch it feel it, it’s hard to understand it,” Khan says. “We as data scientists haven’t done a really great job of explaining AIdriven data technology in simple terms. Telling a story with data or demonstrating actual results is where real power and understanding lies.”
Scale statistical models for actionable models
We often separate our data as factual, asserting “this is what happened.” Neural networks connect the “human decision-making process” to those factual—a simulation practice that helps us make better decisions. Previously, we would look at data sets like demographics, customer behaviors, and such in silos. But when these multiple data sets are connected, it becomes quite evident that no two humans—or customers—are exactly alike.
Technology is now allowing us to understand trends on a factual level and then project outward. Some companies use this key learning to project whether or not a person is likely to suffer a specific affliction in the health realm. It’s also allowing for far more efficacious “if this then what?” scenarios. If a diabetic person takes insulin, controls their diet, then treatment protocol will change. This is enabling highly personalized medicine. But the same processes, principles, and benefits hold in non-health categories as well— encompassing all industries across the board.
Future-proof, Anti-fragile Data Supply Chains
From data connectors to pipelines, data lakes to statistical models, AI to Quantum, visual storyboards to data-driven automation, ML to NLP to Neural Networks, and more, there are highly effective methods for future-proofing your data value chain. The data supply chain is quite complex and, to make it future-proof and non-fragile, it requires thoughtful processing from the point of creation to the point of consumption of actionable insights.
It starts with data acquisition— garnering a wide variety and volume of data from several internal and external sources where data is generated by the millisecond. Once the information is identified and ingested, it needs to be brought to a central point where it can be explored, cleansed, transformed, augmented and enriched, and finally modeled for use toward a purpose. Then comes statistical and heuristic modeling. These models can be of various types using different algorithms, yielding different accuracy levels in different scenarios. Models then need to be tuned and provided an environment for continuous feedback, learning, and monitoring. Finally, the visualization of outcomes is demonstrated by drawing cause-effect relationships that highlight where the most impact happens. This leads to a conclusion on how a set of problems can be solved or opportunities uncovered.
“Most organizations have some data and drive different levels of business process improvement and strategic decisions with it,” Khan notes. “However, few use data to the fullest. The right approach to data valuation and monetization can uncover limitless possibilities, including customer-centricity, operational efficiency, competitive advantage, strategic partnerships, efficient operations, improved profitability, and new revenue streams.”
Multimedia Monetization
Up to now, we have been able to write algorithms, generate immense amounts of numerical or written data and make sense of it. However, a significant amount of data comes as images or voice, which has not been easy to process and manage until recent developments. The applications for the processing of visual and auditory inputs are endless. In fact, retail and finance industries have been early adopters of this technology—and with good reason. They’ve seen costs go down, engagement go up, sales increase, and benefitted from other highly substantial points of monetization.
Now, a large department store can digitize their video data every night and determine that “X” amount of people saw “X” number of jeans, but they had to walk further to get to it. As a result, the department store can put those items closer to the door and walkways to determine if sales increase in kind.
Even the education realm is tapping AI-driven data. The technology is tracking retina movement to discern if kids are engaged amid the remote learning paradigm ushered in by the pandemic. They’re exploring how to measure the retina to determine whether a child is engaged in the lesson.
In radiology, they are starting to convert visual data and track it to gain a deeper understanding of digital images and video. MRIs are better able to track brain tumors—whether they are growing or shrinking and at what rate, and if they are getting darker or lighter in terms of the regions. This kind of AI-driven learning is helping doctors better detect cancer and treat it more rapidly. Video data processing of the human eye can also be used to determine if a person is drunk, fatigued, or even has a disease. Voice machine learning has also keenly evolved. Initially, voice recognition was being utilized to discern if a person was suicidal, which could be accurately predicted by inflection points in a person’s voice. If that person can be captured on video, it is deemed to be about 20 times more accurate.
“All of this possibly had previously demanded a hefty price tag using systems and solutions of yore,” Khan notes. “Today, integrating multiple processes across hybrid multi-cloud environments has made data processing and analytics much more accessible and outsourceable. This negates the need for companies to purchase cost-prohibitive servers and other machine hardware.”
As one of the world’s leading experts on building transparency into supply chains, Khan doesn’t just talk the talk; he’s walked the walk. As a revered marketplace change agent, he’s known for driving business transformation and customer-centric turnaround growth strategies in a multitude of environments. In addition to engineering partnerships with MIT, Khan has successfully led organizational changes and process improvement in markets across the Americas, Europe, Middle East, and Asia.
“New AI solutions and trends will eliminate patchwork processes that cause data, and interpretations thereof, to get lost in translation or, even worse, remain entirely undiscovered,” Khan says. “Next-Gen platforms are solving such problems by executing all functions required to create and govern AI products— single-source systems that pull data, transform, model, tunes and recommend actions with cause-effect transparency.”
For niche players, today’s leading-edge AI technology also aptly provides for vertical industry specialization. “Emerging solutions enable common data models, compliance and interoperability requirements that, in turn, accelerate model validation, refinement, and implementation that’s specific to a given sector or marketplace,” notes Khan. “All of this ultimately drives speed to insights on previously unsolved problems, which reveals untapped opportunities and automates workflow integrated cognitive solutions.”
“Overall, AI is ushering in a new and more sophisticated era of data literacy,” he continues. “It’s a new paradigm founded on automated, comprehensive and holistic data discovery, which is fostering elevated cognitive insights and actionable strategies that positively impact the top and bottom line.”
Perhaps the future mandate for AI should not only focus on becoming smarter, faster, and more accountable than predecessors but bridge the gap between human intuition and data-backed decisions. Doing so will assuredly advance an organization’s ability to transact with utmost trust.
